What is K-means Clustering?
K-means (Macqueen, 1967) is one of the simplest unsupervised learning algorithms that solve the well-known clustering problem. K-means clustering is a method of vector quantization, originally from signal processing, that is popular for cluster analysis in data mining.
K-means Clustering – Example 1:
A pizza chain wants to open its delivery centers across a city. What do you think would be the possible challenges?
- They need to analyze the areas from where the pizza is being ordered frequently.
- They need to understand how many pizza stores has to be opened to cover delivery in the area.
- They need to figure out the locations for the pizza stores within all these areas in order to keep the distance between the store and delivery points minimum.
Resolving these challenges includes a lot of analysis and mathematics. We would now learn about how clustering can provide a meaningful and easy method of sorting out such real-life challenges. Before that let’s see what clustering is.
K-means Clustering Method:
If k is given, the K-means algorithm can be executed in the following steps:
- Partition of objects into k non-empty subsets
- Identifying the cluster centroids (mean point) of the current partition.
- Assigning each point to a specific cluster
- Compute the distances from each point and allot points to the cluster where the distance from the centroid is minimum.
- After re-allotting the points, find the centroid of the new cluster formed.
The step by step process:
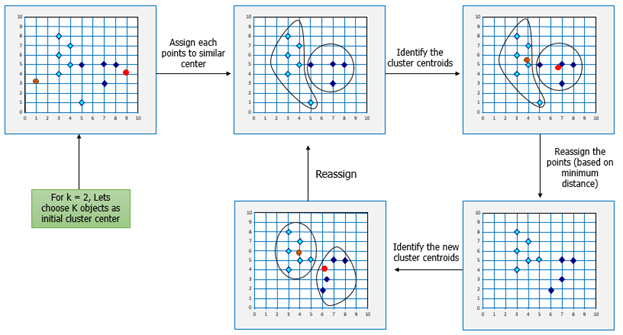