Multi-class classification can be integrated into GANs by conditioning the generator and discriminator on class labels, allowing the model to generate targeted synthetic images for specific classes. Here is the strategies you can follow:
- Conditional Input: Append the class label (e.g., one-hot encoded vector) to the input noise vector for the generator and discriminator.
- Discriminator Conditioning: The discriminator receives both the image and class label, which helps it determine if the picture belongs to the correct class.
- Generator Conditioning: The generator uses both the noise vector and class label to generate class-specific images.
Here is the code snippet you can refer to:
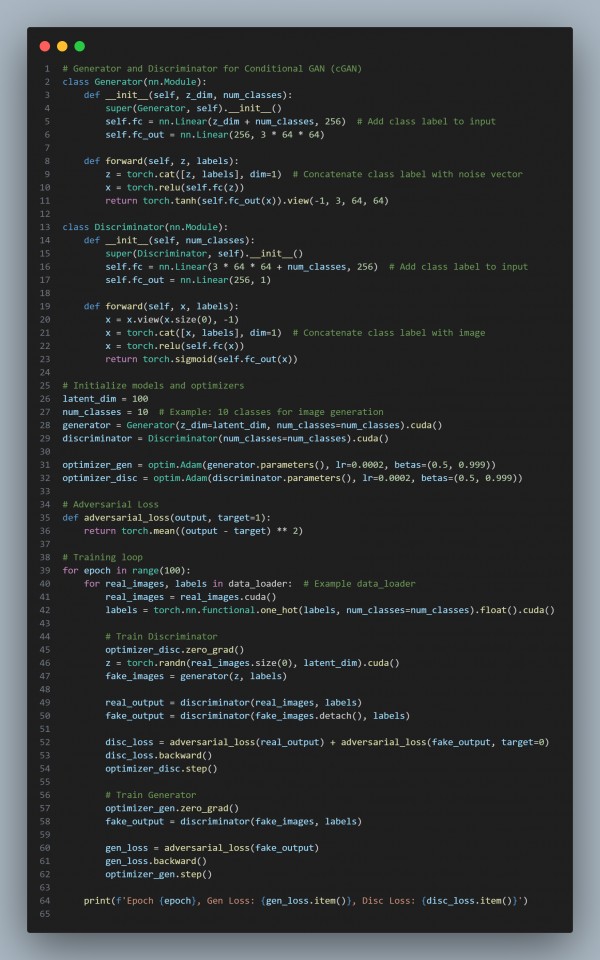
In the above code, we are using the following key features:
- Conditional Input: The class label is concatenated with the noise vector for the generator and with the image for the discriminator.
- Targeted Image Generation: The generator is conditioned to generate images for specific classes based on the input label.
- Multi-Class Classification: The discriminator is trained to distinguish between real and fake images while considering the class label.
- Adversarial Training: Both the generator and discriminator are trained using adversarial loss, with the generator trying to fool the discriminator into classifying its fake images as real.
Hence, by referring to the above, you can integrate multi-class classification into GANs to generate targeted synthetic images.