The RuntimeError: CUDA out of memory occurs when your GPU doesn't have enough memory to store the model, inputs, and intermediate computations. Here's how to resolve it:
- Reduce Batch Size
- Lower the batch size to reduce memory usage.
- Enable Gradient Accumulation
- Simulate a larger batch size by splitting it across iterations.
- Use Mixed Precision Training
- Leverage torch.cuda.amp to minimize memory usage.
- Free Unused Tensors
- Use torch.cuda.empty_cache() to clear unused memory.
- Model Checkpoints
- Save GPU memory by not retaining intermediate states.
- Use Smaller Model or Layers
- Replace heavy layers with lightweight alternatives, e.g., MobileNet instead of ResNet.
Here is the code snippet you can refer to, explaining the following steps:

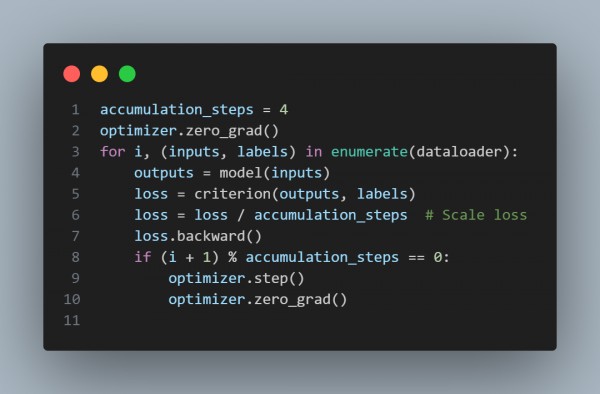
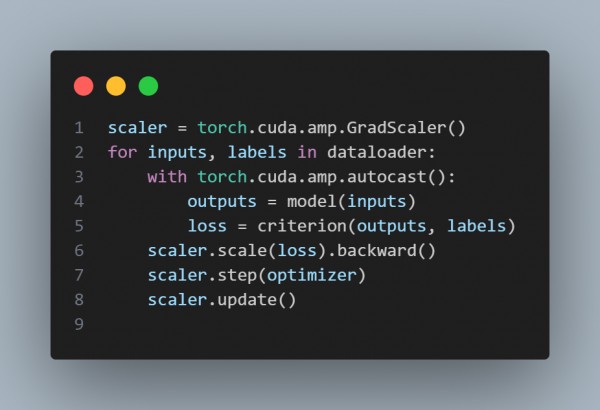
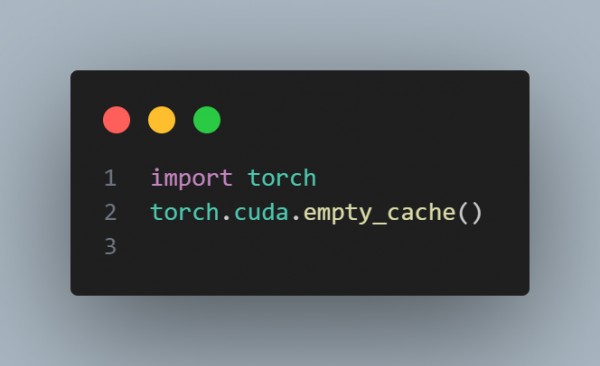

Hence, these techniques can be applied to manage GPU memory efficiently.