You can serve a model using Docker to deploy a GAN for image generation by referring to the following:
- Prepare the GAN Model and Save It
- Save the trained GAN model and necessary preprocessing code.
- Create a Flask App for Serving the GAN
- Write a Python script (app.py) to load the model and handle requests.
- Write a Dockerfile
- Create a Dockerfile to package the Flask app
- Build and Run the Docker Container
- Build the Docker image and run it locally or deploy it to your preferred cloud platform
Here is the code you can refer to:


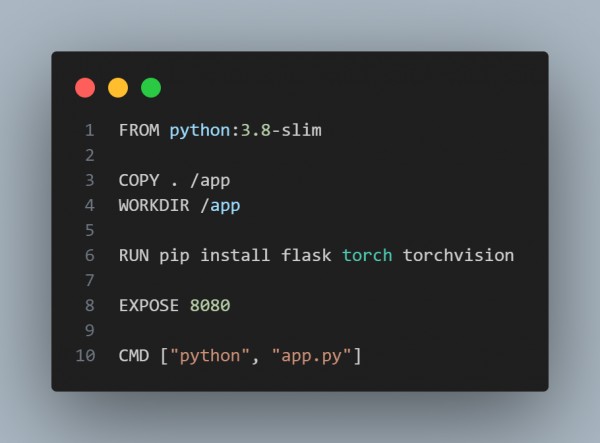

Hence, this setup serves your GAN model via a REST API. Clients can send requests to the /generate endpoint to generate images.