You can effectively evaluate methods for AI-generated content in customer service applications by referring to the following:
- Semantic Similarity Analysis: You can measure how closely AI responses match expected responses using cosine similarity.
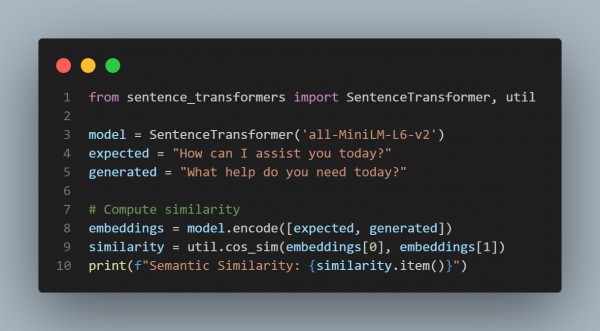
- BLEU or ROUGE Scores: You can evaluate the overlap between AI responses and reference responses.
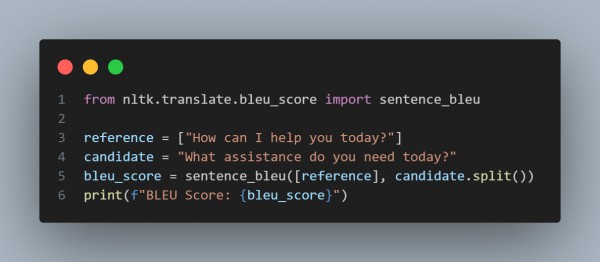
- Sentiment Analysis: You can check if the tone of AI responses aligns with customer service expectations.

- Human Evaluation: You can use Likert scales (1-5) to measure user satisfaction, relevance, and fluency.

In the above code reference, Combining automated metrics (e.g., similarity, BLEU) with human evaluation provides a comprehensive assessment of AI-generated content.
Hence, by using these methods, you can effectively evaluate methods for AI-generated content in customer service applications.