To solve your problem that is in creating a system to generate images based on text discriptions typically involves Text-to-image generation models, such as DALL-E, Midjourney or stable diffusion. Heres a step by step reference you can follow:
Selecting the Model
For generating images from text , you can consider the following models.
- DALL-E 2: A powerful text-to-image model by openai.
- VQGAN+CLIP: Clip understands the relationship between text and images , while VQGAN generates images.
- Stable Diffusion: A recent text-to-image model that can genearte high-quality images efficiently.
Dataset Selection
Now to train such models , you'll need a dataset with paired text discriptions and images. some good datasets include:
- MS-COCO: A large-scale dataset with natural language descriptions and associated images.
- LAION-5B: A dataset specifically created for training models like clip and stable diffusion.
TIPS: You can download dataset from Hugging Face Datasets liberary, which offers direct access to text-image datasets.
- go to the bash and write pip install datasets.
Example to load MS-COCO dataset
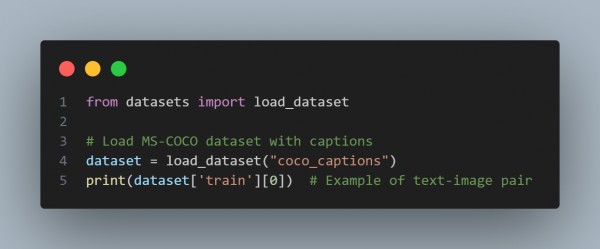
Preprocessing the Data
You need to preprocess both the text and images data for training.
- Text Preprocessing: Tokenize the text descriptions using a tokenizer like GPT,BERT etc.
- Image Preprocessing: Normalize and resize the images to fit the model's input size(e.g.. 256x256 for stable diffusion).
Example for text and image preprocessing:-
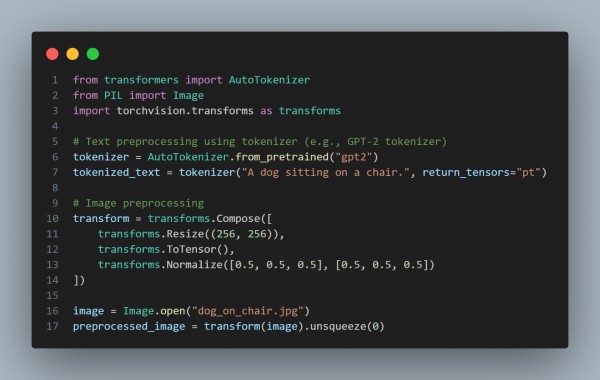
Training the system:
To tarin the system , you'll need to pair your text embeddings (from the tokenizer) with image embeddings .
Heres a basic structure using clip.
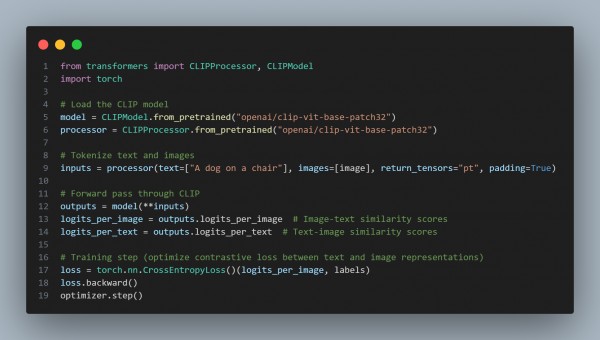
Fine-Tuning the model
Fine-tuning can be performed by training on samllerlearning rates to adjust the pre-trained model for specific dataset.
Here is

Dealing with Potential Challenges:
- Data Imbalance: Ensure diverse descriptions and images to avoid biases.
- Training Stability: You can use techniques like learning rate schedulers , regularization and gradient clipping can help stabilize training.
- Evaluation: Use evaluation metrics like FID to Quantify the quality of generated images.
Inference for Text-to-Image Generation
once trained you can input new text descriptions to generate images:
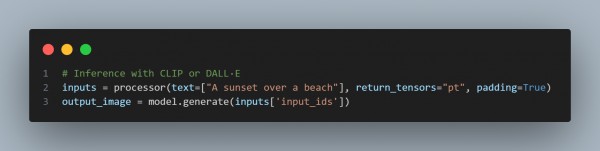
By selecting the appropriate model and dataset ,training it efficiently with techniques like contrastive loss, regularization , and fine-tuning , you can create a high-quality text-to-image generation system.
Related Post: AI image generation aesthetics